You are viewing a preview of...
Rapid Deep Learning-based Flood Losses/Risk Prediction Tool (RAPFLO)
Hierarchical deep neural networks for a rapid and accurate prediction of flood risk
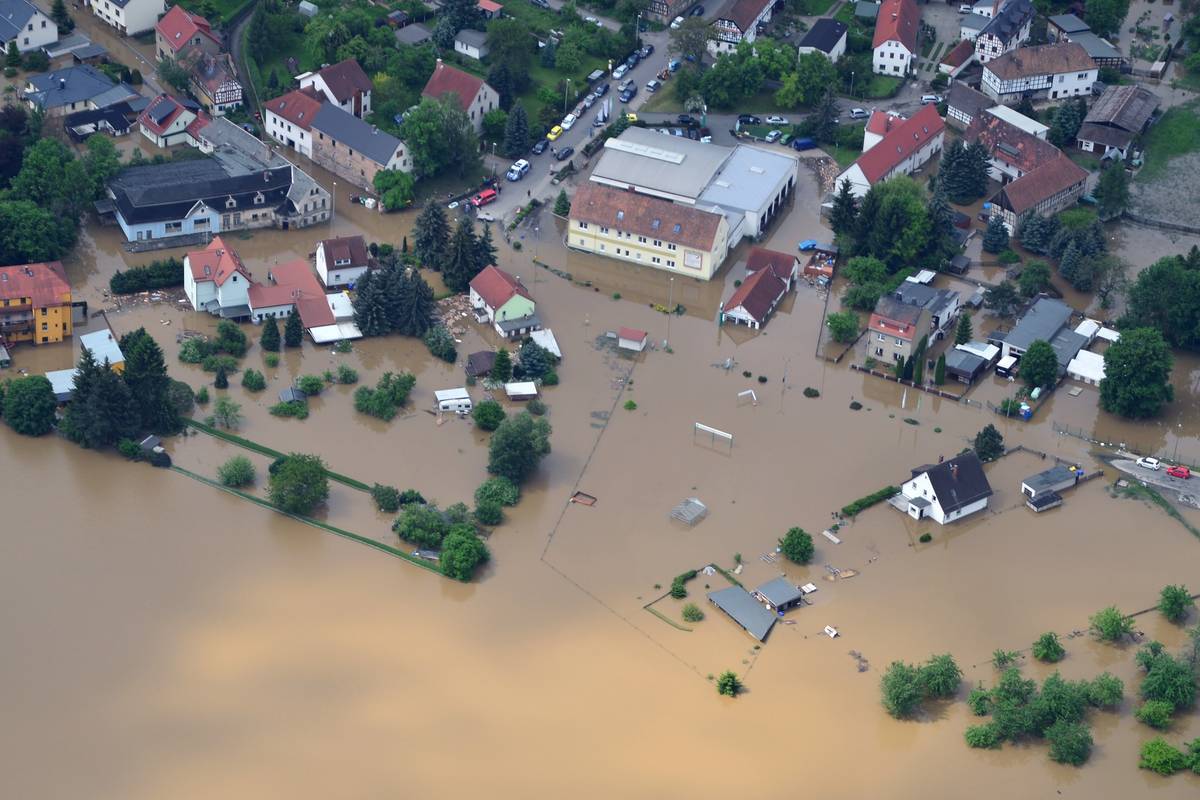
Background
Floods, among the costliest and most destructive weather-related extremes, result in significant casualties, economic losses, and lasting health issues. The complex interplay of meteorological, physical, and anthropogenic factors determines flood risk. System vulnerability, considering social, economic, and physical aspects, plays a crucial role in flood risk assessment. Weighting factors based on expert knowledge or data-driven methods help determine their relative importance. Flood hazard, evaluated through physics-based or data-driven approaches, requires complex hydrologic modeling and hydraulic simulation, often posing computational challenges. Data-driven models correlate location-specific flooding frequency with hydrologic and topographic factors using statistical or machine learning techniques. Deep learning models offer efficient alternatives but require climate model integration for accurate flood risk prediction under climate change. However, in order
Log in or create a free account to continue reading